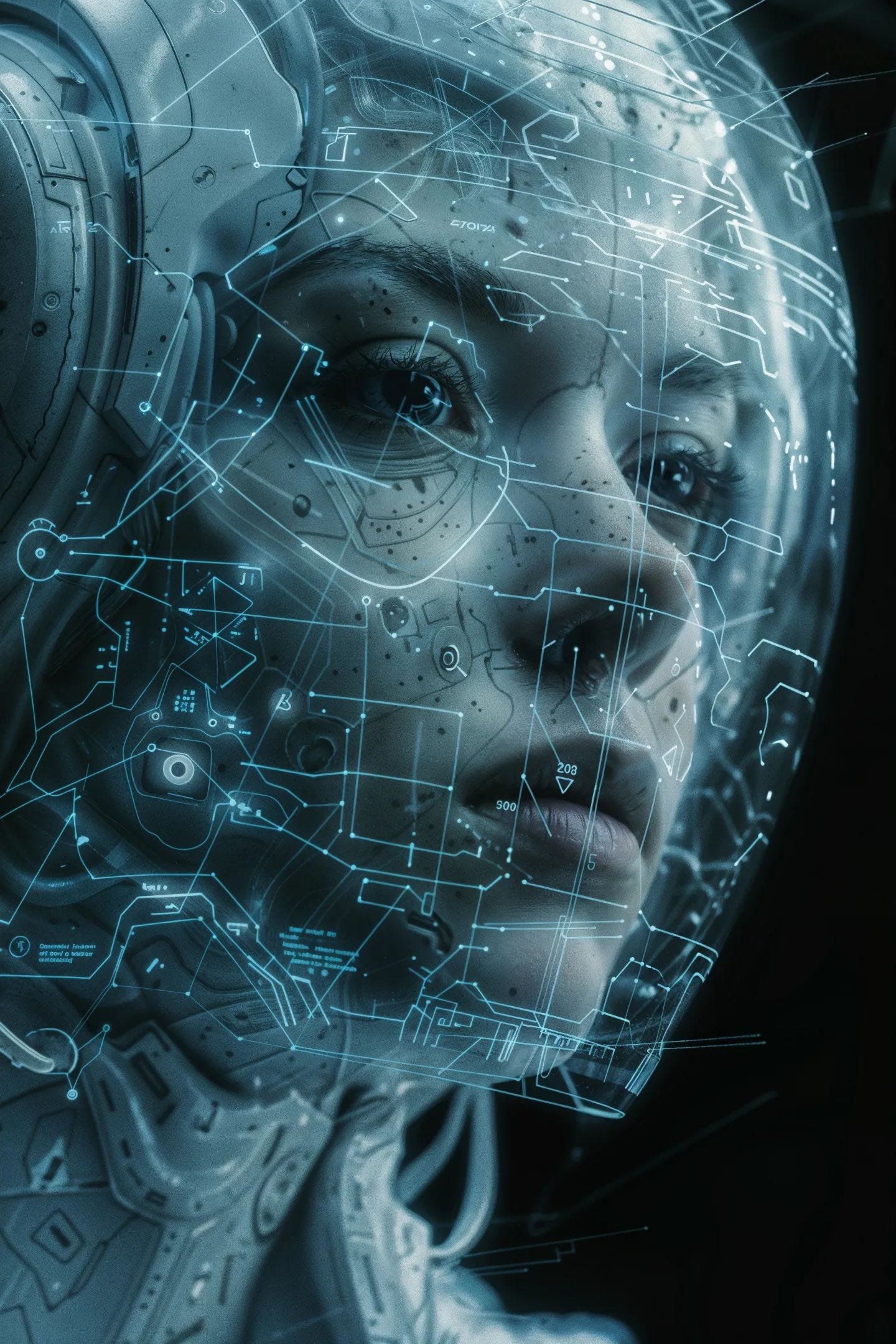
For the first part of this newsletter:
Memories are made by breaking DNA — and fixing it
Nerve cells form long-term memories with the help of an inflammatory response, study in mice finds.
For more: Nature.
"Limitless" Language Models: Exploring the AI Horizon in the Era of Nothing but Blue Skies
Pfizer And AI Drug Discovery
Pfizer Inc. (NYSE: PFE) is an American multinational pharmaceutical and biotechnology corporation headquartered at The Spiral in Manhattan, New York City that was established in 1849 in New York by two German entrepreneurs, Charles Pfizer (1824–1906) and his cousin Charles F. Erhart (1821–1891). Pfizer develops and produces medicines and vaccines for immunology, oncology, cardiology, endocrinology and neurology. The company's largest products by sales are the
Pfizer–BioNTech COVID-19 vaccine ($11 billion in 2023 revenues),
apixaban ($6 billion in 2023 revenues),
a pneumococcal conjugate vaccine ($6 billion in 2023 revenues),
palbociclib ($4 billion in 2023 revenues) and
tafamidis ($3 billion in 2023 revenues).
The company ranks 38th on the Fortune 500 and 39th on the Forbes Global 2000.
Today we are going to see how Pfizer is revolutionizing drug development and pharma marketing by utilizing techbio scientific disciplines, from AI/ML machine and robotics to engineering biology and genomics.
🎾 The Pfizer Innovation Research, PfIRe Lab, is a highly instrumented clinical research facility located in Cambridge, Massachusetts, where researchers are using cutting-edge wearable and environmental sensors to develop and validate novel, patient-centric clinical endpoints, thereby potentially enabling a better understanding of a patient’s disease state and response to therapy. Current studies in the PfIRe lab include:
The ICANGO study that is exploring the use of digital health devices and sensors in healthy participants. And
The MAGIC study that is exploring the use of digital health devices and sensors in healthy children.
🎾 ‘Charlie’, named after Pfizer co-founder Charles Pfizer, is Pfizer’s new AI platform to help with content supply chains and also the entire marketing workbench (With ‘Charlie,’ Pfizer is building a new generative AI platform for pharma marketing). Along with helping with content creation and editing, Charlie also helps with fact-checking and legal reviews. The platform is now in use by hundreds of people in the central marketing team and thousands across the company’s various brands. It’s also being used by agency partners including Publicis Groupe and IPG. Charlie is based on Marcel, the AI platform by Publicis Groupe, and uses training data from a range of Pfizer sources—across treatment categories and products while segmentation models are used to tailor and prioritize messaging—and is powered by a custom ChatGPT and recommendation algorithms. Pfizer is also creating ways to use NLP for queries related to internal research, case studies and performance marketing data.
🎾 Cambridge researchers in collaboration with Pfizer have just published in the journal Nature Chemistry their results regarding a data-driven approach developed and inspired by genomics, where automated experiments are combined with ML to understand chemical reactivity (Probing the chemical ‘reactome’ with high-throughput experimentation data). In particular, they reported the development of a high-throughput experimentation analyser, a robust and statistically rigorous framework, which is applicable to any high-throughput experimentation (HTE) dataset regardless of size, scope or target reaction outcome, which yields interpretable correlations between starting material(s), reagents and outcomes. The ‘reactome’, this AI-powered platform developed by Cambridge and Pfizer, revolutionizes drug design by accurately predicting chemical reactions.
Moreover, they improved the HTE data landscape with the disclosure of 39,000+ previously proprietary HTE reactions that cover a breadth of chemistry, including cross-coupling reactions and chiral salt resolutions. The AI analyser was validated on cross-coupling and hydrogenation datasets, showcasing the elucidation of statistically significant hidden relationships between reaction components and outcomes, as well as highlighting areas of dataset bias and the specific reaction spaces that necessitate further investigation.
🎾 Always in Nature—this time in Nature Communications—Pfizer, the Northwestern University Feinberg School of Medicine, Chicago, IL and the Brigham and Women’s Hospital, Boston, MA presented a ML model for identifying patients at risk for wild-type transthyretin amyloid cardiomyopathy. In particular, they demonstrated that a random forest ML model can identify potential wild-type transthyretin amyloid cardiomyopathy using medical claims data.
They derived a ML model in 1071 cases and 1071 non-amyloid heart failure controls and validated the model in three nationally representative cohorts (9412 cases, 9412 matched controls), and a large, single-center electronic health record-based cohort (261 cases, 39393 controls). They demonstrated that the ML model performs well in identifying patients with cardiac amyloidosis in the derivation cohort and all four validation cohorts, thereby providing a systematic framework to increase the suspicion of transthyretin cardiac amyloidosis in patients with heart failure.