AI-Guided Absorption, Distribution, Metabolism, Excretion, and Toxicity (ADMET) Prediction
An overview of AI/ML tools 🛠️ and startups 🚀 transforming ADMET
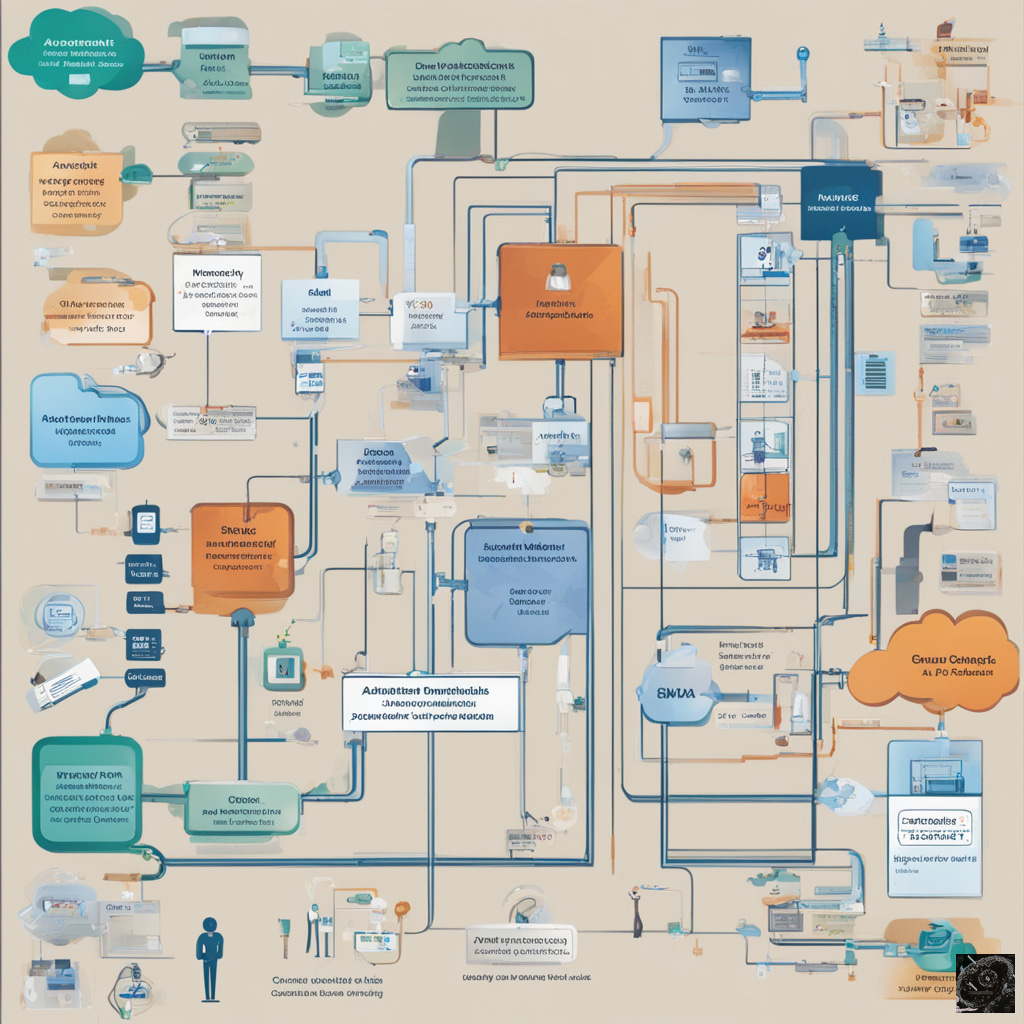
➡️ AI-Guided Absorption, Distribution, Metabolism, Excretion, and Toxicity (ADMET) Prediction: Tools
During screening (How AI is Going to Change Drug Screening Forever
) ensuring good absorption, distribution, metabolism, excretion, and toxicity (ADMET) properties is crucial to a given drug’s success. By exploring ML models, molecular descriptors and structural patterns, precise predictions for blood-brain barrier 🚧 permeability, plasma half-life, solubility, metabolic stability, cytochrome P450 enzyme interactions, potential metabolites, renal excretion, hepatotoxicity assessment and cardiotoxicity can be achieved.➡️ For example, the DeepTox algorithm — that gave outstanding results in the Tox21 Data Challenge, a contest in which the participating groups attempted to computationally predict 12000 environmental chemicals and drugs for 12 different toxic effects in specifically designed assays — first normalizes the chemical representations of the compounds, from which a large number of chemical descriptors are computed and used as the input to ML methods.
The molecular descriptor is the final result of a logic and mathematical procedure which transforms chemical information encoded within a symbolic representation of a molecule into a useful number or the result of some standardized experiment . The descriptors are categorized as:
👉 Static descriptors, that include atom counts, surface areas and the presence or absence of a predefined substructure in a compound. The presence and absence of 2500 predefined toxicophore features (a toxicophore is chemical structure or a portion of a structure that is related to the toxic properties of a chemical), and other chemical features extracted from standard molecular fingerprint descriptors are also calculated. And
👉 Dynamic descriptors, that are calculated in a pre-specified way and despite a potentially infinite number of different dynamic features, the algorithm keeps the dataset within manageable limits.
In typical test cases, the DeepTox pipeline shows good accuracy in predicting the toxicology of compounds, and has already assessed more than 10000 environmental chemicals and drugs for 12+ different toxic effects in explicitly designed assays (DeepTox: Toxicity Prediction using Deep Learning).
➡️ Moreover, quantitative structure-activity relationship (QSAR) based approaches have proven to be very valuable in predicting ADMET. QSAR modeling is a well-known technique that reveals associations between structural characteristics and biological or toxicological activities under the general assumption that similar chemical structures display similar activities (US FDA Experience in the Regulatory Application of (Q)SAR). In fact, QSAR studies are already used extensively and are also increasingly being accepted within the regulatory decision-making process as an alternative to animal tests for toxicity screening. In a recent effort, two QSAR models were developed to predict drug permeability across the blood brain barrier, to assist regulators with abuse liability assessment of drug metabolites or other materials related to drug substances (New developments in regulatory QSAR modeling: a new QSAR model for predicting blood brain barrier permeability).
➡️ A variety of deep neural networks (DNNs for QSAR)—complex computational models such as computer vision and natural language processing—have also generated promising results for QSAR tasks (DeepNeuralNet- QSAR). DNNs can routinely make better predictions than traditional methods, such as random forests, on a diverse collection of QSAR data sets. Moreover, multitask DNN models—those trained on and predicting multiple QSAR properties simultaneously—outperform DNNs trained separately on the individual data sets in many, but not all tasks.
➡️ Then we have the QSOR (Quantitative Structure Odour Relationship) model, namely a subdomain of the QSAR with more complex algorithms in the field of DL and neural networks that has been used for predicting the odour 👃 of a compound based on molecular structure alone.
➡️ Another example is the new MRA Toolbox v.1.0, a web-based toolbox for predicting toxicity of chemical substances. This toolbox is a novel web-based platform including four additive toxicity modules: two conventional (Concentration Addition and Independent Action) and two advanced (Generalized Concentration Addition and Quantitative Structure–Activity Relationship (QSAR)-based Two-Stage Prediction) models. In particular, these quantitative structure-activity relationship (QSAR) based models have proven to be very valuable in predicting physicochemical properties, biological activity, toxicity, chemical reactivity and metabolism of chemical compounds during screening.
➡️ Researchers of the University of Amsterdam, together with researchers at the University of Queensland and the Norwegian Institute for Water Research have presented in the paper From Molecular Descriptors to Intrinsic Fish Toxicity of Chemicals: An Alternative Approach to Chemical Prioritization, a new strategy for assessing the toxicity of chemicals using ML, which is very important since the European and US chemical agencies have listed approximately 800k chemicals that the knowledge of their potential risks to human health and the environment is lacking. The new model tested via 907 experimentally defined 96 h LC50 values for acute fish toxicity, explained ≈90% of the variance in their data for the training set and ≈80% for the test set. This strategy gave a 5-fold decrease in the frequency of incorrect categorization compared to a QSAR regression model. Subsequently, the model was used to predict the toxicity categories of ≈32k chemicals. Then a comparison between the model-based applicability domain (AD) and the training set AD was performed, suggesting that the training set-based AD is a more adequate way to avoid extrapolation when using such models. The better performance of this direct classification model compared to that of QSAR methods makes this approach a viable tool for assessing the hazards and risks of chemicals.
➡️ PBPK mathematical modeling techniques are also widely used in the drug development process in order to predict drug ADME for a range of therapeutics, while in the case of orally administered drugs compartmental absorption and transit (CAT) PBPK models are often used. These models include many details of drug absorption, metabolism and transport in the gastrointestinal tract, which is often divided into discrete segments, each of which may account for drug in various states. Implementations of such models include the ACAT (advanced compartmental and transient) model, part of the proprietary software GastroPlus (by SimulationPlus), and the OpenCAT model, developed de novo using the open source software GNU MCSim.
Both ACAT or OpenCAT require solving complex differential equations that may make implementation difficult in certain workflows. One approach to address these limitations is to capture the essential features of PBPK simulation predictions within a trained ML model, that takes as an input drug-specific information and would output essential PK parameters as a stage in a multi-element analysis pipeline. In fact, the aim of this work Adapting physiologically-based pharmacokinetic models for machine learning applications was to explore the feasibility of this approach using OpenCAT as the underlying PBPK model. In particular, the model was tested for drug formulations spanning a large range of solubility and absorption characteristics, and was evaluated for concordance against predictions of OpenCAT and relevant experimental data. In general, the values predicted by the ML models were within 20% of those of the PBPK model across the range of drug and formulation properties. However, summary PK parameter predictions from both the ML model and full PBPK model were occasionally poor with respect to those derived from experiments, suggesting deficiencies in the underlying PBPK model.
➡️ In this study Prediction of Small-Molecule Developability Using Large-Scale In Silico ADMET Models, they report a novel DL approach that leverages large-scale predictions of ∼100 ADMET assays to assess the potential of a compound to become a relevant drug candidate. The resulting score, termed the bPK score, substantially outperformed previous approaches and showed strong discriminative performance on data sets where previous approaches did not.
➡️ The new SwissADME is a new web tool that gives free access to a pool of fast yet robust predictive models for physicochemical properties, pharmacokinetics, drug-likeness and medicinal chemistry friendliness, among which in-house proficient methods such as the:
The BOILED-Egg model that delivers a rapid, intuitive, easily reproducible yet statistically unprecedented robust method to predict the passive gastrointestinal absorption and brain access of small molecules useful for drug discovery and development,
iLOGP, a simple, robust, and efficient description of n-Octanol/Water partition coefficient for drug design using the GB/SA approach, and
The Bioavailability Radar that is used for a rapid assessment of drug-likeness in which six physicochemical properties are taken into account: lipophilicity, size, polarity, solubility, flexibility and saturation.
UK launches £100M AI Life Sciences fund to treat incurable diseases.
“AI can help us solve some of the greatest social challenges of our time. AI could help find novel dementia treatments or develop vaccines for cancer. That’s why today we’re investing a further £100 million to accelerate the use of AI on the most transformational breakthroughs in treatments for previously incurable diseases.” UK’s Prime Minister Rishi Sunak
➡️ Databases for AI-Guided Absorption, Distribution, Metabolism, Excretion, and Toxicity (ADMET) Prediction
The Key Databases for ADMET property prediction are:
💡 ChemIDplus, is a free web search system that provides access to the structure and nomenclature authority files used for the identification of chemical substances cited in National Library of Medicine (NLM) databases, including the TOXNET system (a computerized system of files oriented to toxicology and related areas),
💡 ToxRefDB, contains in vivo study data from over 5900 guideline or guideline-like studies, allowing scientists and the interested public to access thousands of curated animal toxicity testing results, which is a great resource for many retrospective and predictive toxicology applications,
💡 BindingDB, is an online database of measured binding affinities focused primarily on the interaction of drug target proteins with small drug-like molecules. BindingDB contains 2.8M data for 1.2M Compounds and 9.2K Targets. Of those, 1,297K data for 594K Compounds and 4.5K Targets were curated by BindingDB curators. BindingDB is a FAIRsharing resource,
💡 DrugBank, is a free comprehensive database of drugs and drug targets (over 500,000 drugs & drug products) that contains different chemicals and target information for each drug,
💡 PubChem, is an integrated chemistry database that includes small to large molecules with structure, physical properties, bioactivity, patents, etc, and
💡 ChEMBL, is a curated database of bioactive drug-like small molecules that mainly covers 2D structures, calculated properties and bioactivity (115M Compounds, 309M Substances, 293M Bioactivities, 36M Literature and 38M Patents).
Future House, a philanthropically-funded moonshot focused on building an AI Scientist in Biology.
Future House—a philanthropically-funded moonshot focused on building an AI Scientist, with a 10-year mission to build semi-autonomous AI tools for scientific research, to accelerate the pace of discovery and to provide world-wide access to cutting-edge scientific, medical, and engineering expertise—was launched last week.
Focused on biology, through its impact on medicine, food security and climate, Future House is an independent, non-profit research organisation, headquartered in San Francisco, hiring right now for AI researchers, wet lab biology researchers and other roles.
➡️ AI-Guided Absorption, Distribution, Metabolism, Excretion, and Toxicity (ADMET) Prediction: Startups 🚀
🔦 ADMET Predictor 11—by SimulationsPlus a premier developer of drug development software as well as a leading provider of both preclinical and clinical pharmacometric consulting services for regulatory submissions and quantitative systems pharmacology models for drug-induced liver injury and nonalcoholic fatty liver disease—is the latest version of the flagship ML platform for ADMET modeling and its extended capabilities for data analysis, metabolism prediction and AI-driven drug design. In this video, Eric Jamois, Director of Business Development and the VP of ADMET Cheminformatics, David Miller, will walk you through the new features of AP11, covering:
Greatly improved pKa model incorporating large contribution of experimental data from pharmaceutical partners (pKa is the acid dissociation constant (Ka) of a solution is. The pKa value is one method of determining an acid's strength).
New and updated models of CYP inhibition (the cytochrome P450 (CYP) enzyme family is the most important enzyme system catalyzing the phase 1 metabolism of pharmaceuticals and other xenobiotics such as herbal remedies and toxic compounds in the environment. The inhibition and induction of CYPs are major mechanisms causing pharmacokinetic drug-drug interactions).
New functionality for performing 3D similarity screens based on shape and pharmacophore features. And
Significant enhancements to the AI-driven drug design module.
🔦 The German startup molab.ai provides a step-change in drug and compound discovery through robust ADMET predictions and a compound optimisation suite. They have a highly accurate ADMET property predictions with reliable confidence indicators providing actionable recommendations for novel molecules—with very robust performance on uncharted chemical space, outperforming physics-based models as well as other AI/ML solutions. They offer also, a Compound optimisation suite for suggestions for novel, alternative molecular structures optimised for improved binding affinity, desirable ADMET properties and synthetic accessibility. Molab.ai is looking for highly skilled candidates to fill the following vacant position of Machine Learning Intern to contribute to the development of their ML pipeline, by driving their own projects, and working closely with their experienced ML Engineering and Research colleagues.
🔦 Genesis Therapeutics (California, US, 2019) spun out of Stanford University has created the industry's most advanced molecular AI platform—GEMS, Genesis Exploration of Molecular Space—which integrates DL and molecular simulations for property prediction and language models for molecular generation. Genesis has also developed Dynamic PotentialNet as a proprietary approach to predict potency, selectivity and ADMET, that provides GEMS with unprecedented speed and accuracy in property predictions, including for data-poor and previously undruggable targets.
Key components of its platform have been rigorously tested against current state-of-the-art methods in a collaboration between Stanford university and a top-five pharma company. So far, they achieved a step-change improvement predicting 20+ different ADMET properties (Improvement in ADMET Prediction with Multitask Deep Featurization). In August 2013, Genesis has closed an oversubscribed $200 million round of Series B financing, co-led by a U.S.-based life-sciences-focused investor, along with returning investor Andreessen Horowitz (a16z) Bio + Health which led the company’s seed financing.
🔦 DeepCure (Boston, Massachusetts, US, 2018) is a startup that uses DL to discover small molecule therapeutics. MolDBTM, their proprietary database for virtual screening has over a trillion unique molecules. After identifying the most promising small molecule per target, they optimise for ADMET properties. Their patent-pending molecular generation tool, MolGen™, leverages state of the art deep reinforcement learning to construct synthesizable molecules that efficiently capture all causal interactions for binding and selectivity while maintaining the desired ADME-tox profile (DeepPropR™). On January, 4, 2023, Biosero, a developer of laboratory automation solutions, and DeepCure announced their collaboration on an advanced, fully automated chemistry synthesis platform to accelerate drug discovery.
An overview of AI’s increasing traction in drug discovery and development in 2023
When Pharma met Artificial Intelligence (substack.com)
🔦 Cortex Discovery in Germany has a modern ML approach to rapidly and confidently screen a vast number of compounds and to identify high quality compounds with higher chances of success in progressing through preclinical drug discovery. They offer the following services:
Screen large libraries or virtually generate de novo compounds,
Predict on-target interactions (hit discovery),
Predict off-target interactions (polypharmacology), and
Drug metabolism and toxicity, ADMET, profiling:
Physicochemical (Log P, Solubility in water, Solubility in DMSO at 10 mM)
ADE (Solubility in FaSSIF, PAMPA permeability, Caco-2 A→B permeability, Blood brain barrier permeation, Fraction unbound in plasma, Half-life in plasma, Half-life in liver microsome)
Toxicity (Cytotoxicity in various cell lines, Genotoxicity, hERG inhibitor)
Metabolism (CYP, AhR, CAR, PXR, CSTO1, ALDH1A1).
🔦 The Chinese startup CarbonSilicon offers a drug discovery workflow leveraging AI-generated content (AIGC), self-supervised pre-training, reinforcement learning and physics-based modeling. The startup’s activity prediction solution, Inno-Docking, provides complete protein preparation, ligand preparation and intelligent setting of docking parameters. Additionally, Inno-Rescoring features AI-scoring functions to evaluate protein-ligand binding affinity. CarbonSilicon’s comprehensive druggability assessment involves three computational modules:
Inno-ADMET for ADMET properties,
ChemFH to filter frequent hit compounds and
Inno-SA to predict substructure-related toxicity.
🔦 Iktos (Paris, France, 2016) is developing a proprietary and innovative solution based on DL generative models, which enables using existing data to design new molecules. To guide the molecular generator in the infinity of the chemical space, they have introduced fitness functions that the algorithm must optimise. Those functions are predictors, specifically built from a project’s data set: molecules, measurements from the assays, activity, selectivity and ADMET. Iktos has developed so far: DockAI (docking), Makya (de novo design), Spaya (retrosynthesis) and SpayaAPI (a scoring tool for virtual molecule libraries).
Finally, this year,
Curreio, a Japanese company specializing in structural analysis of biomolecules using cryo-electron microscopy, combined Iktos’ de novo generative design technology with its state-of-the-art cryo-EM platform,
Kissei Pharmaceutical Co., a Japanese pharmaceutical company specialized in the field of urology, kidney-dialysis and unmet medical needs, has introduced Makya as a Service Platform for its AI-driven drug discovery platform, and
Iktos has won the “Big Data & AI for Good” prize at the 12th edition of the Big Data & AI Paris Congress on 25th September.
For more AI Startups for ADMET Prediction.
Until next time,